ORGANOID CULTURES IN SILICO: TOOLS OR TOYS?
written by Dr. Torsten Thalheim, Dr. Gabriela Aust, Dr. Joerg Galle
Interdisciplinary Institute for Bioinformatics (IZBI), Leipzig University, Härtelstr. 16–18, 04107 Leipzig, Germany; Department of Surgery, Research Laboratories, Leipzig University, Liebigstraße 20, 04103 Leipzig, Germany
Bioengineering2023, 10(1), 50; https://doi.org/10.3390/bioengineering10010050
(This article belongs to the Special Issue Material and Engineering-Based Approaches for Organoids)
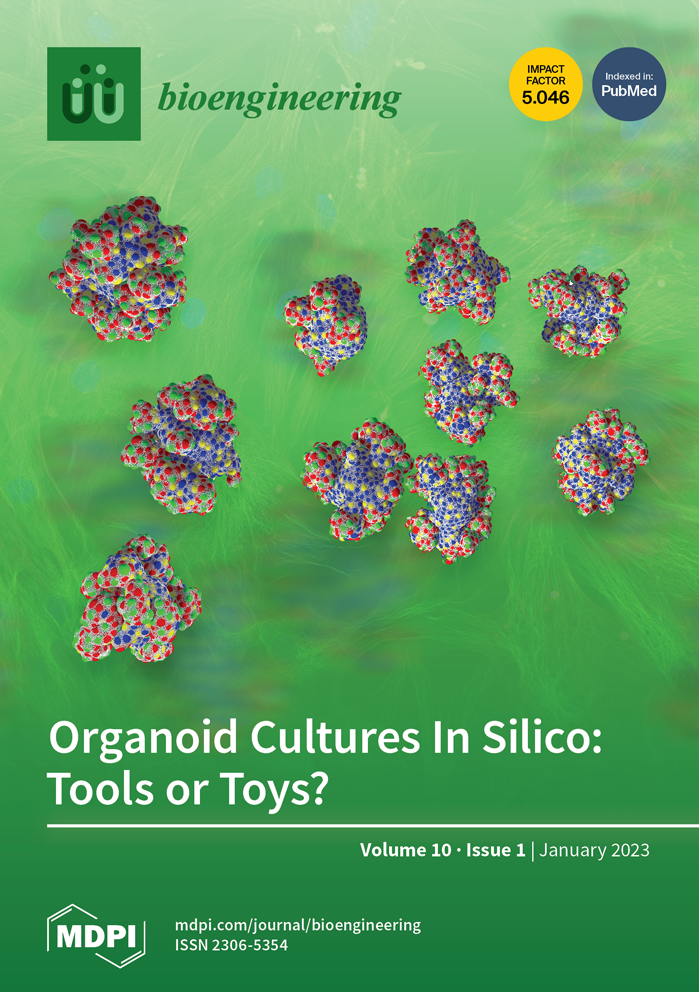
ABSTRACT
The implementation of stem-cell-based organoid culture more than ten years ago started a development that created new avenues for diagnostic analyses and regenerative medicine. In parallel, computational modelling groups realized the potential of this culture system to support their theoretical approaches to study tissues in silico. These groups developed computational organoid models (COMs) that enabled testing consistency between cell biological data and developing theories of tissue self-organization. The models supported a mechanistic understanding of organoid growth and maturation and helped linking cell mechanics and tissue shape in general. What comes next? Can we use COMs as tools to complement the equipment of our biological and medical research? While these models already support experimental design, can they also quantitatively predict tissue behavior? Here, we review the current state of the art of COMs and discuss perspectives for their application.
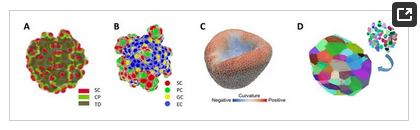
INTRODUCTION
Organoids are 3D self-organizing multicellular aggregates that differentiate in vitro into functional cell types, recapitulating the structure and function of the tissue of origin. They can be established from embryonic (ESC), induced-pluripotent (iPSC) or adult stem cells (ASCs). While ESC- and iPSC-derived organoids involve stepwise differentiation protocols that resemble SC regulation during gastrulation and organogenesis, ASC-derived organoids require media supplemented with signal pathway activators and/or inhibitors that support SC maintenance under tissue homeostasis. Landmarks in the organoids field were ESC-derived cortical tissues and organoids derived from ASCs of the small intestine. Meanwhile, organoids exist for many other tissues including heart, liver, lung, mammary gland, pancreas, prostate, skin, stomach and more. An organoid cell atlas has been envisioned. In our review, we focus on organoids that resemble fetal, adult or tumor tissue. We do not include gastruloids that resemble tissues at early embryonic development and tumor spheroids that emulate aspects of 3D tumor mass expansion only.
In order to gain mechanistic insight into organoid formation and to support culture design computational organoid models (COMs), i.e., in silico counterparts of organoids, have been developed in the last decade. The approaches are as diverse as the computational methods typically used to describe 3D cell aggregates (Figure 1). Differential equation-based continuum models describe cell aggregates based on spatio-temporal density distributions of cell types (Figure 1A). In contrast, individual cell-based models represent each cell as a 3D object. This model class includes models where cells of defined shape can move, proliferate and differentiate into several lineages (Figure 1B), but also vertex-based approaches where cells have very flexible shape enabling a better approach to the mechanics of dense tissue (Figure 1C). In order to take cell composition of aggregates directly from experiments, Voronoi tessellation can be used to define cell shape based on the distribution of the cell nuclei (Figure 1D). Notably, this method has been applied in 2D COMs only. Advantages and disadvantages of these methods are summarized in Table 1. COMs based on these approaches typically address questions about tissue self-organization. Frequently, they build on computational models of the tissue of origin. COMs with simpler, often fixed geometry were introduced to inform experimentalist how to optimize oxygen and nutrient transport or drug delivery.
…
SUMMARY
Current COMs explain specific phenomena of organoid growth, shape formation or cell composition (Figure 5). In order to simulate organoid-based disease models, it might not be necessary to consider all these aspects. However, including different levels of SC regulation into COMs seems to be essential. Their specific requirements mutually confine the space of solutions of the model and can eventually result in a few stable states. Screening the COM’s parameter space will then inform about the phenomena, which can be covered by the model and for which parameters they occur. In case these parameters depend on the environment, one can start a drug response simulation. The above considerations argue for COMs that combine multiscale feedback-models of SC differentiation (e.g., including transcriptional regulation by epigenetics and metabolic regulation of epigenetics) with models of differentiated cells, which describe their lineage specific metabolism and mechanics. Thereby, individual cells are probably best represented by vertex models because they straightforwardly enable considering feedback of tissue mechanics on lineage specification and allow inclusion of collective motion. Such a COM needs to be further combined with reaction-advection-diffusion models to calculate local concentrations of nutrients and metabolites.
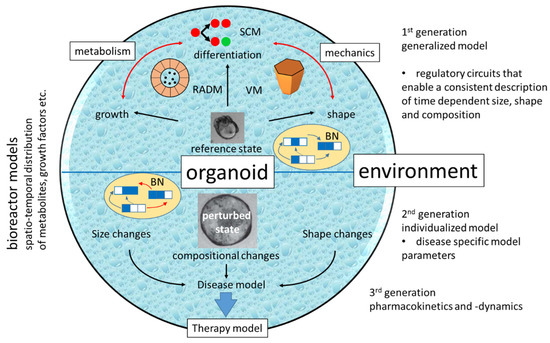
Figure 5.In silico organoids. Currently available are 1st generation COMs for different tissue. However, they often focus on a single aspect such as organoid differentiation, shape formation and limited growth. Accordingly, they use different approaches such as individual cell-based SC models (SCM), vertex models (VM) or reaction-advection-diffusion model (RADM). Missing are COMs combining these aspects in a core regulatory circuit, e.g., a Boolean network (BN), with a limited number of regulatory states. In 2nd generation COMs, individual model parameters and/or regulatory links are changed to simulate a disease model. Its behavior under therapy can then be tested combining it with a ‘bioreactor model’ describing the organoid’s environment.
An obstacle to this approach might be a problem inherent to computational tissue modelling. The strength of a model approach is that it can provide simple hypotheses on tissue organization that are verifiable by experiments. For this purpose, models often use a level of abstraction that omits major variation. However, this kind of ‘noise’, i.e., these small variations are what most clinical applications are based upon. Here, the question arises whether COMs should reach an ever-increasing level of complexity to describe them. Beside the risk of implementation faults that might remain hidden, while increasing their complexity, the COMs would lose their most important feature of providing direct insight into tissue self-organization. A one-to-one representation of organoids in silico thus does not seem to be desirable. Approaches using methods of artificial intelligence might be better suited for such mapping. However, because transcription circuits can evolve in ways that preserve their output, complex states are not necessarily uniquely defined, resulting in limitations of such extensions as well.
A current trend in the application of organoids includes immune cell co-culture and microbiome studies. Related COMs can be expected in the near future. They will need to consider the interaction of organoid cells with other cell types or pathogens. Whether these additional agents should be modeled individually is not clear.
Problems not addressed in COMs so far are: (i) mechanism of in vitro cell adaptation, (ii) changes associated with long-term organoid culture and (iii) effects of culture derived from aged individuals. A trend for enhanced in vitro compared to in vivo transformation has been shown not only for organoids originating from mice suffering from mismatch repair deficiency, but also for organoids derived from normal mice, although at a lower level. Moreover, long-term epigenetic drifts are found in organoid culture, seemingly changing the competence to adapt to stress. Thus, in silico modeling of organoid safety aspects might be a further challenge in the field.
In this review, we have not discussed technical questions of in silico modelling of organoids, as their implementation and computational power. Agent-based software frameworks for COMs have been discussed by Montes-Olivas et al. Clearly, according to the different levels of model complexity, the simulation effort in central processing unit (CPU-) time very much varies between the model classes. For complex individual cell-based models, it might reach the limits of currently available resources, limiting their broad application. Cell simulation techniques dedicated to running on graphics processing units might help to solve these problems.
CONCLUSION
In the past decade, computational modelling of tissue organization has very much benefited from organoid research. The new quality of data available on regulatory processes has enabled scientists to validate hypotheses on fundamental principles of tissue growth and self-organization and thus to improve in silico tissue modelling. While these studies extend our knowledge on cell metabolism, cell biomechanics and SC organization, the links between these aspects of tissue organization are still largely unknown. However, this knowledge is essential to understand self-organization of organoids, which is a prerequisite for simulation of pharmacological interventions and other perturbations of normal tissue function. Accordingly, support of clinical applications of organoids by COM approaches is mostly limited to pharmacokinetics. Thus, whether COMs will become essential tools in organoid based disease and therapy modeling remains to be seen in future. Currently, individual cell-based COMs are more or less nice toys for impactful visualization of related research questions.
to read the full article at MDPI, please click here: https://www.mdpi.com/2306-5354/10/1/50