Machine learned-based visualization of the diversity of grapevine genomes worldwide and in Armenia using SOMmelier
written by K. Magaryan, M. Nikogհosyan, A. Baloyan, H. Gasoyan, E. Hovhannisyan, L. Galstyan, T. Konecny, A. Arakelyan and H. Binder,
1 Research Group of Plant Genomics, Institute of Molecular Biology of National Academy of Sciences RA, Yerevan 0014, Armenia
2 Department of Genetics and Cytology, Yerevan State University, Yerevan 0025, Armenia
3 Armenian Bioinformatics Institute (ABI), Yerevan 0014, Armenia
4 Bioinformatics Group, Institute of Molecular Biology Institute of National Academy of Sciences RA, Yerevan 0014, Armenia, Yerevan 0014, Armenia
5 Interdisciplinary Centre for Bioinformatics, University of Leipzig, 04107 Leipzig, Germany
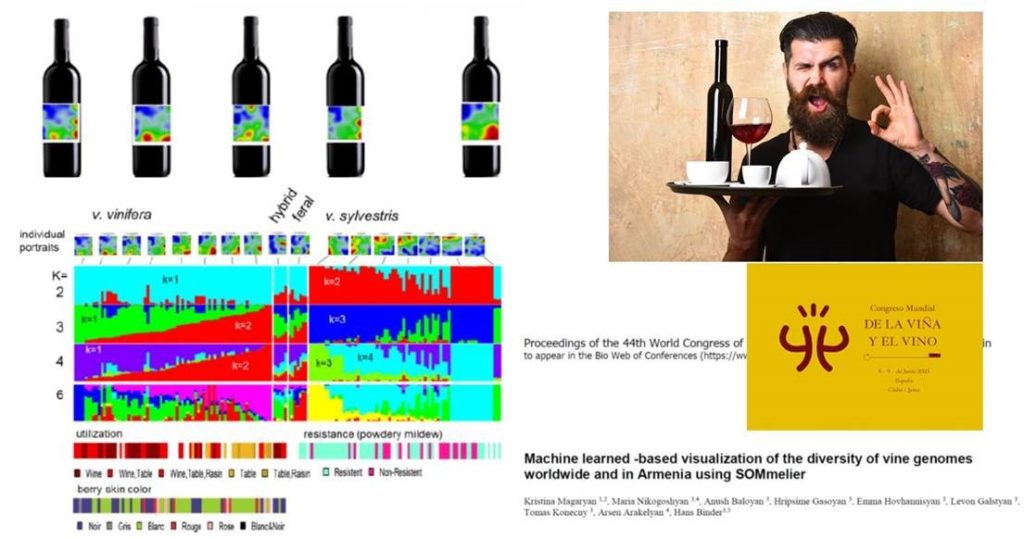
ABSTRACT
In the proposed study three major issues have been addressed: Firstly, the diversity of grapevine accessions worldwide and particularly in Armenia, a small country located in the largely volcanic Armenian Highlands, is incredibly rich in cultivated and especially wild grapes; secondly, the information hidden in their (whole) genomes, e.g., about the domestication history of grapevine over the last 11,000 years and phenotypic traits such as cultivar utilization and a putative resistance against powdery mildew, and, thirdly machine learning methods to extract and to visualize this information in an easy to percept way. We shortly describe the Self Origanizing Maps (SOM) portrayal method called “SOMmelier” (as the vine-genome “waiter”) and illustrate its power by applying it to whole genome data of hundreds of grapevine accessions. We also give a short outlook on possible future directions of machine learning in grapevine transcriptomics and ampelogaphy.
INTRODUCTION
The grapevine is one of the earliest domesticated fruit crops and has been widely cultivated and prized for its fruit and wine. According to the recent study [1] the roots of domestication were found deep in the Pleistocene, ending almost 11.5 thousand years ago (ya) in the region, where Armenian Highland is existed. Armenia is considered an ancient origin of grapevine domestication and wine-making, which is confirmed by remains of wild and cultivated grapes and wine-producing facilities found at archaeological sites of the country. The diverse climatic conditions, unique geography and existence of wild grapes were the main drivers in the formation of extensive diversity of cultivated varieties and the promotion of wine-making [2].
In the recent decade whole genome studies of grapevine genetic resources using high-throughput sequencing technologies have generated novel knowledge about the evolution of vine traits, genetic diversity, phylogenetic relatedness and historical origin, phenotype associations and migration paths of the vines.There has been a rapid growth in the quality and quantity of data for grapevine genomes, but methods to interrogate this data are limited. At the same time, machine learning and artificial intelligence methods are revolutionising data analysis. Presented research applied machine learned-based visualization and analysis of grapevine genomic data by SOMmelier method to gain a greater understanding of grapevine genomes, their diversity, function and evolution [3].
Self-organizing neural networks mainly referred to as self-organizing maps (SOMs) were introduced by T. Kohonen in the beginning of 1980’s, who presented them as “a new, effective software tool for the visualization of high-dimensional data” [4]. The methods has been further developed into a molecular portrayal method complemented by comprehensive downstream analysis options including different visualization options, knowledge mining and feature selection tasks [5]. It has been applied mainly to different omics data in the human disease context (see, e.g., [6,7]) and recently was applied to a collection of SNP vine genome data [3]. Here we shortly introduce the method, illustrate its power by applying it to worldwide grapevine genomes to reconstruct dissemination of viticulture, discuss the impact of wild and cultivated grapevines collected in Armenia and finallypresent first results of whole genome analyses using SOMmelier of Armenian grapevine gene pool.
. . .
CONCLUSION
Whole genome data on thousands of grapevine accessions open novel perspectives in viticulture. Machine learning and, particularly, SOMelier molecular portrayal in combination with other bioinformatics methods offers interesting options for their intuitive analysis and understanding in terms of mutual similarities as well as of their functional impact. The detailed study of the richness of Armenian genetic resources is in the focus of our research addressing the history of grapevine cultivation, resistance against fungal diseases and environmental stress in the context of climate change.
We acknowledge the support given by FAST (Foundation of Armenian Science and Technology) in the frame of the ADVANCE program and project 21T-1F076, SC of RA.
to read the full article, click here: https://www.bio-conferences.org/articles/bioconf/full_html/2023/13/bioconf_oiv2023_01009/bioconf_oiv2023_01009.html