PROJECTION OF HIGH-DIMENSIONAL GENOME-WIDE EXPRESSION ON SOM TRANSCRIPTOME LANDSCAPES
writen by Maria Nikoghosyan, Henry Loeffler-Wirth, Suren Davidavyan, Hans Binder and Arsen Arakelyan
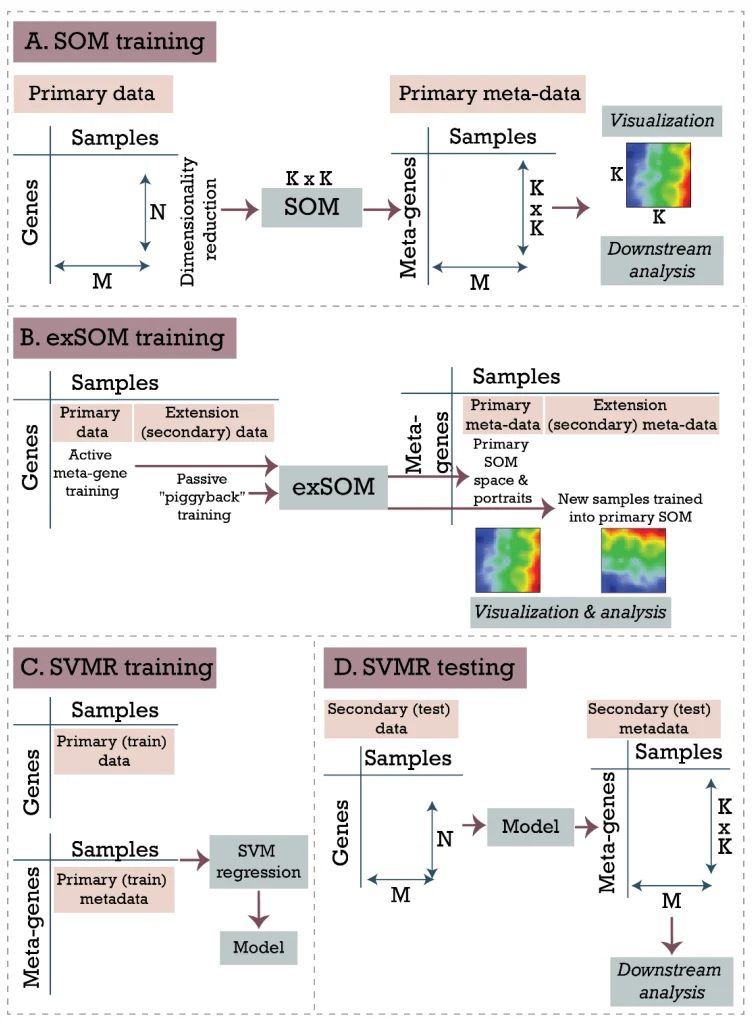
The self-organizing maps portraying has been proven to be a powerful approach for analysis of transcriptomic, genomic, epigenetic, single-cell, and pathway-level data as well as for “multi-omic” integrative analyses. However, the SOM method has a major disadvantage: it requires the retraining of the entire dataset once a new sample is added, which can be resource- and time-demanding. It also shifts the gene landscape, thus complicating the interpretation and comparison of results.
To overcome this issue, we have developed two approaches of transfer learning that allow for extending SOM space with new samples, meanwhile preserving its intrinsic structure. The extension SOM (exSOM) approach is based on adding secondary data to the existing SOM space by “meta-gene adaptation”, while supervised SOM portrayal (supSOM) adds support vector machine regression model on top of the original SOM algorithm to “predict” the portrait of a new sample.
Both methods have been shown to accurately combine existing and new data. With simulated data, exSOM outperforms supSOM for accuracy, while supSOM significantly reduces the computing time and outperforms exSOM for this parameter. Analysis of real datasets demonstrated the validity of the projection methods with independent datasets mapped on existing SOM space. Moreover, both methods well handle the projection of samples with new characteristics that were not present in training datasets.
CONCLUSIONS:
In this paper, we described options for extending SOM-based high-dimensional transcriptomic data portraying with additional, independent samples. The two extension approaches presented enable overcoming the main limitation of SOM machine learning, namely, that adding samples or complete datasets changes the intrinsic primary structure of primary SOM. Both exSOM and supSOM demonstrated their utility in overcoming this drawback. Both methods have their advantages and disadvantages: while exSOM seems more accurate, supSOM is time-efficient. From the methodical side, the novelty of the study is provided by the combination of previous SOM portrayal neural network machine learning with extrapolation of metagene values for novel samples using additive transfer learning approaches which transfer novel data into a multidimensional space obtained from previously collected data. The novel methods considerably widen the application range of SOM portrayal because they not only make computations more effective but, especially, because they enable usage of always analyzed data space for novel samples. Analysis of inflammatory disease and cancer datasets demonstrated the validity of the projection methods with independent datasets mapped on existing SOM space.
Moreover, we showed that the methods well handle the projection of samples with new characteristics that were not present in training datasets (see the “inflammatory bowel disease response to infliximab” section of the Results).Thus, we demonstrated that SOM extension methods (exSOM and supSOM) can remarkably extend the usage scenarios of SOM “molecular data portrayal” approaches.
to read the complete article, please click here: https://www.mdpi.com/2673-7426/2/1/4/htm