INTEGRATED MULTI-OMICS MAPS OF LOWER-GRADE GLIOMAS
written by Hans Binder Maria Schmidt, Lydia Hopp Suren Davitavyan Arsen Arakelyan and Henry Loeffler-Wirth
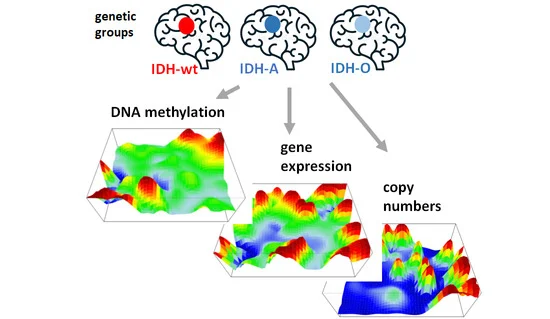
SUMMARY
Data from multiple omics domains were increasingly generated in large-scale tumour studies to enhance our understanding of molecular mechanisms of cancer. We present an integrated cartography of three omics layers combining the transcriptome, methylome, and genome (copy number variations) into a unique mapping scheme which enabled us to decipher functional links within and between the omics domains. Application to lower grade gliomas reveals distinct networks governed either by methylation or copy number variations, both affecting transcriptomics modes of cell activity. The integrated maps provide an intuitive view on tumour heterogeneity across the omics layers distinguishing, e.g., astrocytoma- and oligodendroglioma-like glioma types. In a wider sense, multi-omics cartography deciphers the effect of different omes on tumour phenotypes and their molecular hallmarks with individual resolution.
ABSTRACT
Multi-omics high-throughput technologies produce data sets which are not restricted to only one but consist of multiple omics modalities, often as patient-matched tumour specimens. The integrative analysis of these omics modalities is essential to obtain a holistic view on the otherwise fragmented information hidden in this data. We present an intuitive method enabling the combined analysis of multi-omics data based on self-organizing maps machine learning. It “portrays” the expression, methylation and copy number variations (CNV) landscapes of each tumour using the same gene-centred coordinate system. It enables the visual evaluation and direct comparison of the different omics layers on a personalized basis. We applied this combined molecular portrayal to lower grade gliomas, a heterogeneous brain tumour entity. It classifies into a series of molecular subtypes defined by genetic key lesions, which associate with large-scale effects on DNA methylation and gene expression, and in final consequence, drive with cell fate decisions towards oligodendroglioma-, astrocytoma- and glioblastoma-like cancer cell lineages with different prognoses. Consensus modes of concerted changes of expression, methylation and CNV are governed by the degree of co-regulation within and between the omics layers. The method is not restricted to the triple-omics data used here. The similarity landscapes reflect partly independent effects of genetic lesions and DNA methylation with consequences for cancer hallmark characteristics such as proliferation, inflammation and blocked differentiation in a subtype specific fashion. It can be extended to integrate other omics features such as genetic mutation, protein expression data as well as extracting prognostic markers.
CONCLUSIONS
Multi-omics SOM cartography allows for disentangling the diversity of regulatory modes of cell functions in terms of easy-to-interpret gene-centric data landscapes. They visualize aberrant changes related to complex diseases such as cancer. The method is not restricted to the triple-omics data used here. It can be extended to integrate other omics features such as genetic mutation or protein expression data. “Phenotype” association with survival of the patients and other clinical characteristics potentially extends the visualization options of the method. Due to the growing use of multi-omics data, we expect that these options will become important for future progress in cancer bioinformatics.
click here to read the complete article: https://www.mdpi.com/2072-6694/14/11/2797